The Internet of Things (IoT) refers to the ever-growing network of physical objects connected over IP networks. With the proliferation of IoT devices in everyday life - from home automation to wearables - comes several challenges in both network communications and security. The ACI's Internet of Things Research Lab (IoTRL) enables us to explore the communication that occurs between these objects and other internet-enabled devices and systems. This helps inform how we think about cybersecurity in the future and how we can improve network architectures and protocols to promote better security while enabling data-driven insights from interconnected systems.
Use Cases
- Multi-modal data generation, collection, and processing

- IoT device hacking and exploration
- Edge AI/ML training, testing and experimentation
- Network/sensor data visualization and monitoring
Tools
Our current tool suite consists of several open-source platforms such as Elasticsearch, Kibana, Grafana, InfluxDB, Security Onion, and Wireshark, along with some Government off-the-shelf monitoring tools such as Lava, Dagger, and Galaxy.

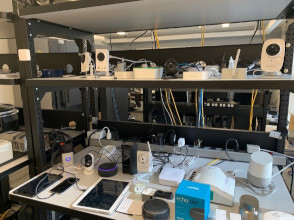
Devices
We currently have networked 10+ IoT devices to generate, capture, and store sensor (audio, video, image, text, etc.) and network data with more being added to model smart-home scenarios
Datasets
We have utilized our facilites to create state of the art datasets, found below
ACI IoT Network Traffic Dataset 2023
Nack, E., McKenzie, M. & Bastian, N. (2024). ACI-IoT-2023: A Robust Dataset for Internet of Things Network Security Analysis. Proceedings of the 2024 IEEE Military Communications Conference, pp. 1-6. IEEE.
Zhang, L., Riem, J., Chen, J., Mackay, H., Lan, T., Bastian, N. & Adam, G. (2024). Multi-Memristor Based Distributed Decision Tree Circuit for Cybersecurity Applications. IEEE Transactions on Circuits and Systems, 71(8): 3526-3537.
Y. A. Farrukh, S. Wali, I. Khan and N. D. Bastian, Detecting Unknown Attacks in IoT Environments: An Open Set Classifier for Enhanced Network Intrusion Detection, MILCOM 2023 - 2023 IEEE Military Communications Conference (MILCOM)
Berenbeim, A., Bierbrauer, D., Cruickshank, I., Thomson, R., & Bastian, N. (2023). Applications of Certainty Scoring for Machine Learning Classification in Multi-modal Contexts. TechRxiv.
Chen, J., Zhang, L., Riem, J., Adam, G., Bastian, N., & Lan, T. (2023). Explainable Learning-Based Intrusion Detection Supported by Memristors. Proceedings of the 2023 IEEE Conference on Artificial Intelligence, pp. 195-196. IEEE.
Willeke, M., Bierbrauer, D. & Bastian, N. (2023). Data-Efficient, Federated Learning for Raw Network Traffic Detection. Proceedings of the 2023 SPIE Conference on Artificial Intelligence and Machine Learning for Multi-Domain Operations Applications V (125380Y), pp. 125380Y-1 – 125380Y-17, SPIE Defense + Commercial Sensing (Volume: 12538).
Bastian, N., Jha, S., Tabuada, P., Veeravalli, V. & Verma, G. (2023). Principles of Robust Learning and Inference for Internet of Battlefield Things. In Robert Douglass, Keith Gremban, Ananthram Swami and Stephan Gerali (Ed.), Internet of Things for Defense and National Security (pp. 119-128). Wiley-IEEE Press.
Bierbrauer, D., De Lucia, M., Reddy, K., Maxwell, P. & Bastian, N. (2023). Transfer Learning for Raw Network Traffic Detection. Expert Systems with Applications, 211(118641): 1-10.
Abdelzaher, T., Bastian, N., Jha, S., Kaplan, L., Srivastava, M. & Verravalli, V. (2022). Context-Aware Collaborative Neuro-Symbolic Inference in Internet of Battlefield Things. Proceedings of the 2022 IEEE Military Communications Conference, pp. 1053-1058. IEEE.
Cobb, A., Jalaian, B., Bastian, N. & Russell, S. (2021). Robust Decision-Making in the Internet of Battlefield Things Using Bayesian Neural Networks. Proceedings of the 2021 Winter Simulation Conference (Ed. Kim et al.), pp. 1-12. IEEE.
Cobb, A., Jalaian, B., Bastian, N. & Russell, S. (2021). Toward Safe Decision-Making via Uncertainty Quantification in Machine Learning. In William Lawless, Ranjeev Mittu, Donald Sofge, Thomas Shortell and Tom McDermott (Eds.), Systems Engineering and Artificial Intelligence (pp. 379-399). Springer, Cham.
Division Chief, Data and Decision Sciences | Principal Research Scientist, Army Cyber Institute
Senior AI Researcher, Army Cyber Institute
Research Scientist, Army Cyber Institute
-
AI Researcher, Army Cyber Institute
Technical Product Manager, Army Cyber Institute